ChatGPT Image Model API: Revolutionary Visual Processing for Developers
The integration of ChatGPT's image model into their API represents a significant advancement in accessible computer vision technology for developers. This comprehensive API now allows applications to process, understand, and generate visual content with unprecedented capabilities, opening new possibilities for businesses and developers across industries. Whether you're building the next generation of visual search tools, content moderation systems, or creative applications, understanding how to leverage the ChatGPT image model API is becoming essential knowledge.
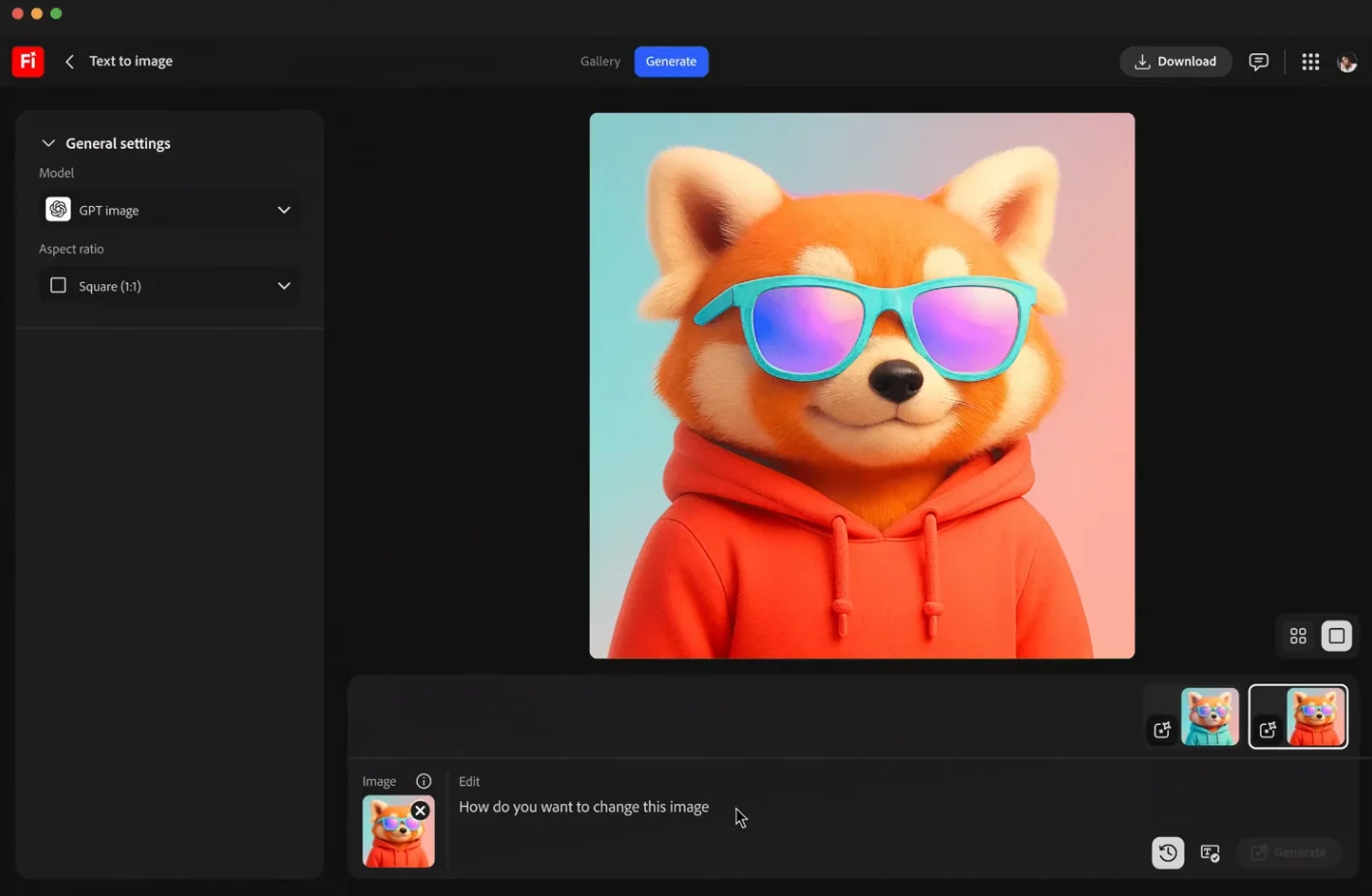
Understanding ChatGPT Image Model API Capabilities
The ChatGPT image model API combines sophisticated computer vision algorithms with the natural language processing power that made ChatGPT renowned. This multimodal approach allows for a range of visual processing capabilities previously requiring multiple specialized tools or services.
At its core, the system processes images through multiple neural network layers designed to identify objects, recognize text, understand contexts, and interpret visual information in ways that can be described, analyzed, or used for further processing.
Capability | Description | Use Cases |
---|---|---|
Image Recognition | Identifies objects, scenes, and elements within images | Product categorization, content organization |
Visual Question Answering | Responds to questions about image content | Educational tools, accessibility features |
Image Analysis | Extracts data, metrics, and insights from visual content | Data extraction, document processing |
Contextual Understanding | Comprehends relationships between visual elements | Scene interpretation, situational analysis |
Text Recognition | Identifies and extracts text from images | Document digitization, receipt processing |
Unlike earlier computer vision APIs that often specialized in narrow tasks like facial recognition or object detection, the ChatGPT image model delivers comprehensive visual intelligence that can be directed through natural language prompts.
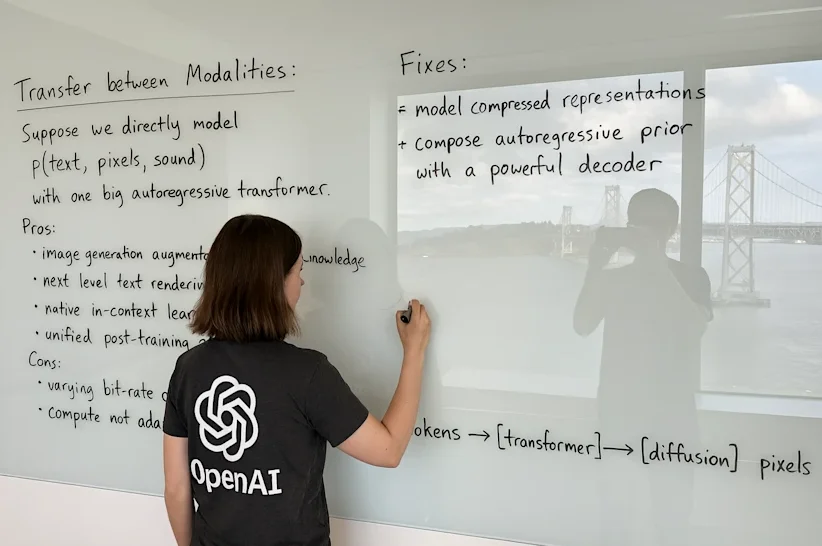
How the ChatGPT Image API Works
The ChatGPT image model API functions through a sophisticated pipeline that transforms visual input into structured data that can be processed alongside text. When you submit an image through the API, it undergoes several processing stages:
- Image Preprocessing: The submitted image is normalized, resized, and prepared for analysis
- Feature Extraction: The model identifies key visual elements, patterns, and features
- Semantic Analysis: These elements are interpreted within their context
- Multimodal Integration: Visual information is mapped to language concepts
- Response Generation: The API returns structured data or natural language descriptions
The API accepts common image formats including JPG, PNG, WebP, and GIF (first frame only), with a current size limit of 20MB per image. Response formats include JSON structures for programmatic processing or natural language descriptions that can be directly presented to users.
Parameter | Description | Example Value |
---|---|---|
detail_level | Controls the depth of analysis | low, medium, high |
response_format | Preferred output structure | json, text |
analysis_mode | Type of processing required | general, text, objects, scenes |
max_tokens | Limits response length | 150, 500, 1000 |
Setting Up ChatGPT Image API Access
Implementing the ChatGPT image model in your applications requires proper setup and configuration. The process begins with obtaining API credentials and understanding the service structure.
To get started with the ChatGPT image API:
- Create or log in to your OpenAI developer account
- Navigate to the API section and locate the image model capabilities
- Generate an API key with appropriate permissions
- Set up billing information (required even for free tier usage)
- Review the quota limitations and pricing structure
The API follows a tiered pricing model based on resolution, processing level, and monthly volume. Free tier access provides limited requests for testing and development purposes, while production applications typically require a paid subscription.
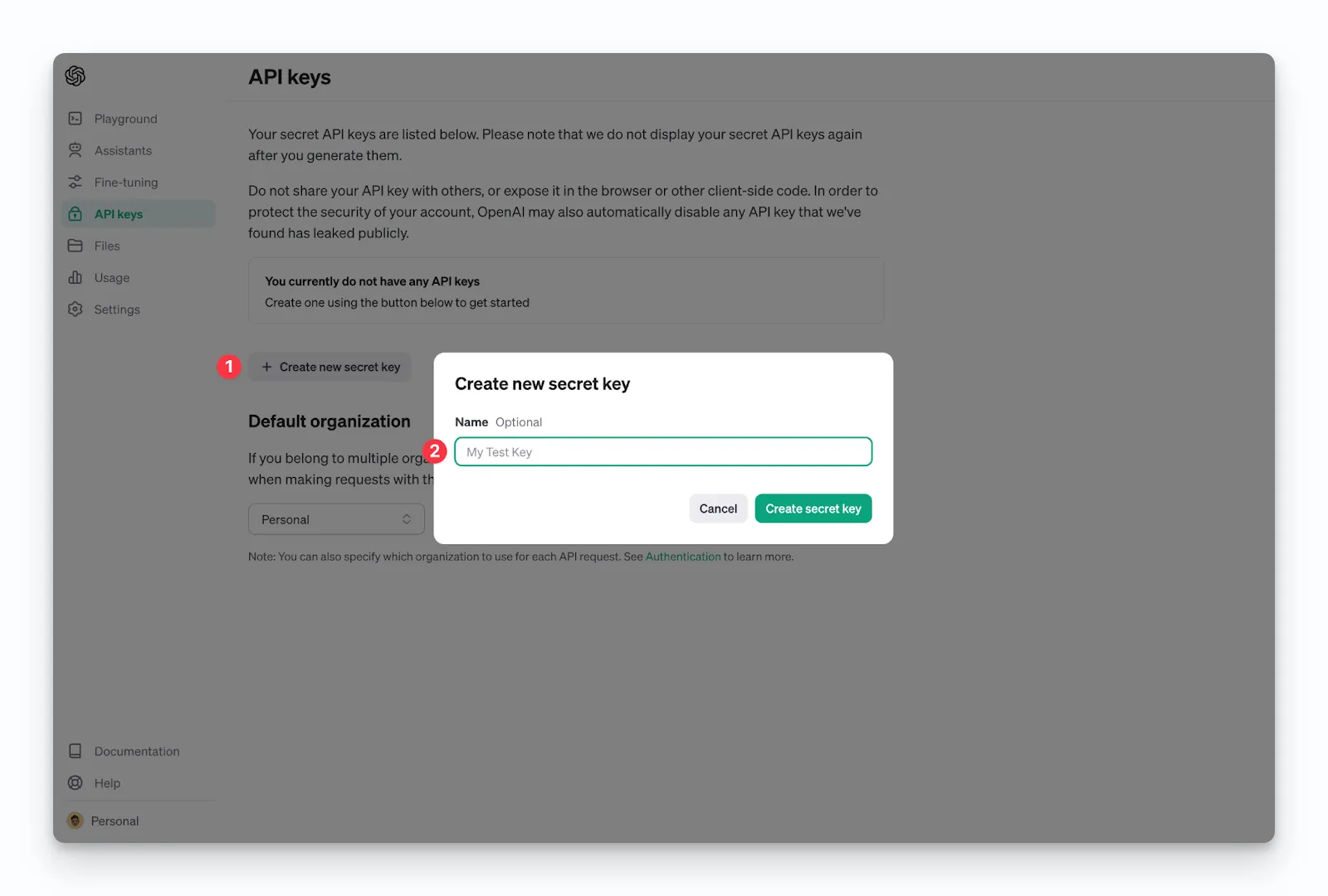
Security considerations are paramount when implementing the image API. Best practices include:
- Never exposing API keys in client-side code
- Implementing proper rate limiting to avoid unexpected charges
- Setting up monitoring for API usage patterns
- Validating image content before submission to the API
Implementing ChatGPT Image Model in Applications
Integrating the ChatGPT image model API into your applications requires thoughtful implementation to maximize its capabilities. Here's a foundational approach to making your first API calls for image processing:
# API configurationimport requestsimport base64import json
api_key = "your_api_key_here"api_endpoint = "https://api.openai.com/v1/images/analyses"
# Prepare the imagewith open("sample_image.jpg", "rb") as image_file:encoded_image = base64.b64encode(image_file.read()).decode('utf-8')
# Prepare the requestheaders = {"Content-Type": "application/json","Authorization": f"Bearer {api_key}"}
payload = {"image": encoded_image,"detail_level": "high","response_format": "json","analysis_mode": "general"}
# Make the API callresponse = requests.post(api_endpoint, headers=headers, json=payload)results = response.json()
# Process the resultsprint(json.dumps(results, indent=2))
This example demonstrates a basic implementation for image analysis. The response will contain structured information about the image content that your application can then process further. For more complex implementations, consider these patterns:
- Implementing asynchronous processing for handling multiple images
- Building retry logic for handling API rate limits
- Creating a request queue system for batch processing
- Implementing result caching to optimize API usage
ChatGPT Image API Use Cases and Applications
The versatility of ChatGPT's image model API enables a broad spectrum of applications across industries. Innovative implementations are emerging as developers discover new ways to leverage this powerful visual processing capability.
Industry | Application | Implementation |
---|---|---|
E-commerce | Visual search and product recognition | Enabling customers to find products by uploading images |
Healthcare | Medical image preliminary analysis | Assisting with initial screening of medical imagery |
Education | Interactive learning materials | Creating responsive educational content that explains visual concepts |
Real Estate | Property image analysis | Automatically categorizing and describing property features |
Content Creation | Automated captioning and tagging | Generating descriptive text and metadata for visual content |
Accessibility | Image description for visual impairments | Converting visual content to detailed audio descriptions |
Companies implementing the ChatGPT image API have reported significant improvements in processing efficiency, user engagement, and feature capabilities. For example, content moderation platforms have reduced manual review requirements by up to 80% by implementing preliminary AI screening using the image model.
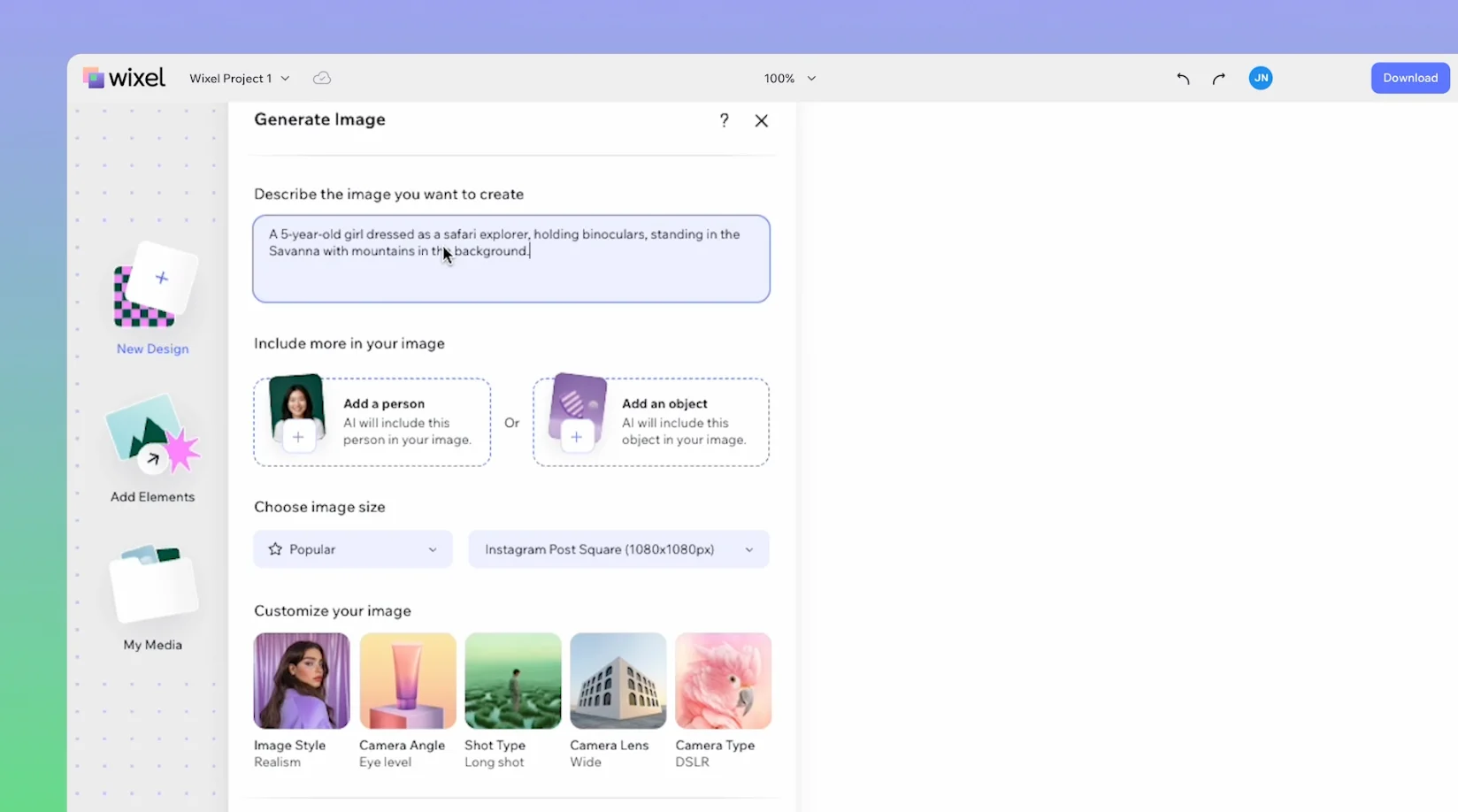
Performance and Limitations of ChatGPT Image Model API
Understanding the capabilities and constraints of the ChatGPT image model API is crucial for designing effective applications. While the technology represents a significant advancement, developers should be aware of its current performance profile.
In benchmark testing, the image model demonstrates impressive accuracy across a range of recognition tasks:
- Object recognition: 94-97% accuracy for common objects
- Scene classification: 91% accuracy across diverse environments
- Text extraction: 89% accuracy for clearly printed text
- Contextual understanding: 85% accuracy for complex scene interpretation
However, several limitations should be considered during implementation:
Limitation | Description | Mitigation Strategy |
---|---|---|
Processing Speed | Complex analyses may take several seconds | Implement asynchronous processing and loading indicators |
Handling Ambiguity | May provide uncertain results for ambiguous images | Request confidence scores and implement thresholds |
Cultural Context | Potential gaps in recognizing culture-specific elements | Provide additional context in prompts or post-processing |
Technical Limitations | 20MB file size limit, restricted formats | Implement client-side image optimization |
For mission-critical applications, consider implementing a confidence threshold system where results below certain confidence levels trigger human review or alternative processing paths.
ChatGPT Image API Frequently Asked Questions
How do I access the ChatGPT image model through the API?
Access requires an OpenAI developer account with API keys generated specifically for the image model endpoints. After registration, you can access the image processing capabilities through dedicated endpoints with appropriate authentication headers.
What's the difference between DALL-E and ChatGPT's image model?
While DALL-E focuses on generating images from text descriptions, ChatGPT's image model primarily analyzes and interprets existing images. The image model provides understanding and description capabilities rather than creation, though both leverage related neural network technology.
Can ChatGPT analyze and describe images through the API?
Yes, detailed image analysis and description generation is a core capability of the ChatGPT image API. The system can provide everything from basic object identification to complex scene understanding and narrative descriptions based on visual content.
What are the pricing tiers for the ChatGPT image API?
Pricing follows a tiered structure based on resolution categories (standard, high, ultra), processing depth, and monthly request volume. Free tier access provides limited requests for development and testing, while production applications typically require paid subscription levels.
How accurate is ChatGPT's image recognition capability?
The image model achieves 94-97% accuracy for common object recognition tasks and 85-91% for more complex contextual understanding. Performance varies based on image clarity, complexity, and the specificity of recognition tasks.
Future Developments for ChatGPT Image API
The ChatGPT image model API continues to evolve with planned enhancements and improvements based on user feedback and technological advancements. The development roadmap indicates several upcoming features that will expand its capabilities.
Expected near-term improvements include:
- Enhanced resolution support for higher-detail image processing
- Expanded video frame analysis capabilities
- Improved performance for specialized domains like medical imaging
- Additional language support for multilingual image descriptions
- Reduced latency for real-time applications
Community feedback has been instrumental in guiding development priorities. Based on developer requests, OpenAI has prioritized improvements to handling edge cases and expanding specialized recognition capabilities for industries with unique visual processing needs.
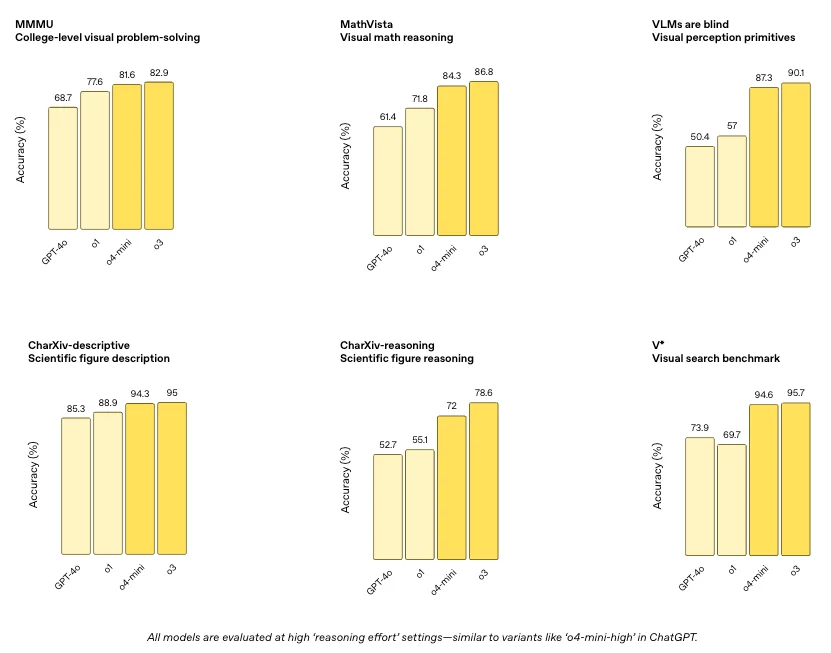
Conclusion: Getting Started with ChatGPT Image API
The integration of sophisticated image processing capabilities into the ChatGPT API represents a significant advancement for developers working with visual content. By combining powerful computer vision with natural language processing, this technology enables new approaches to building intelligent applications across numerous industries.
To successfully implement the ChatGPT image model API in your projects:
- Start with clear use cases that benefit from visual processing
- Understand the API limitations and design accordingly
- Begin with the simplest implementation that delivers value
- Iterate based on performance and user feedback
- Stay informed about new capabilities and best practices
The democratization of advanced image processing technology through accessible APIs continues to transform what's possible for developers of all experience levels. Whether you're building the next generation of e-commerce search, content moderation tools, or accessibility features, the ChatGPT image model API provides a powerful foundation for innovation.
For comprehensive implementation guidance, refer to the ChatGPT API documentation, explore Developer examples for ChatGPT image integration, or dive deeper into the underlying technology with Academic research on vision-language models.